Quickly reading very large tables as dataframes
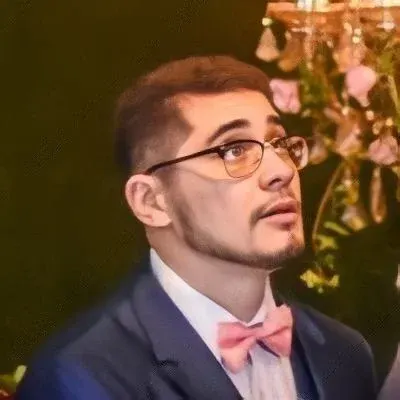
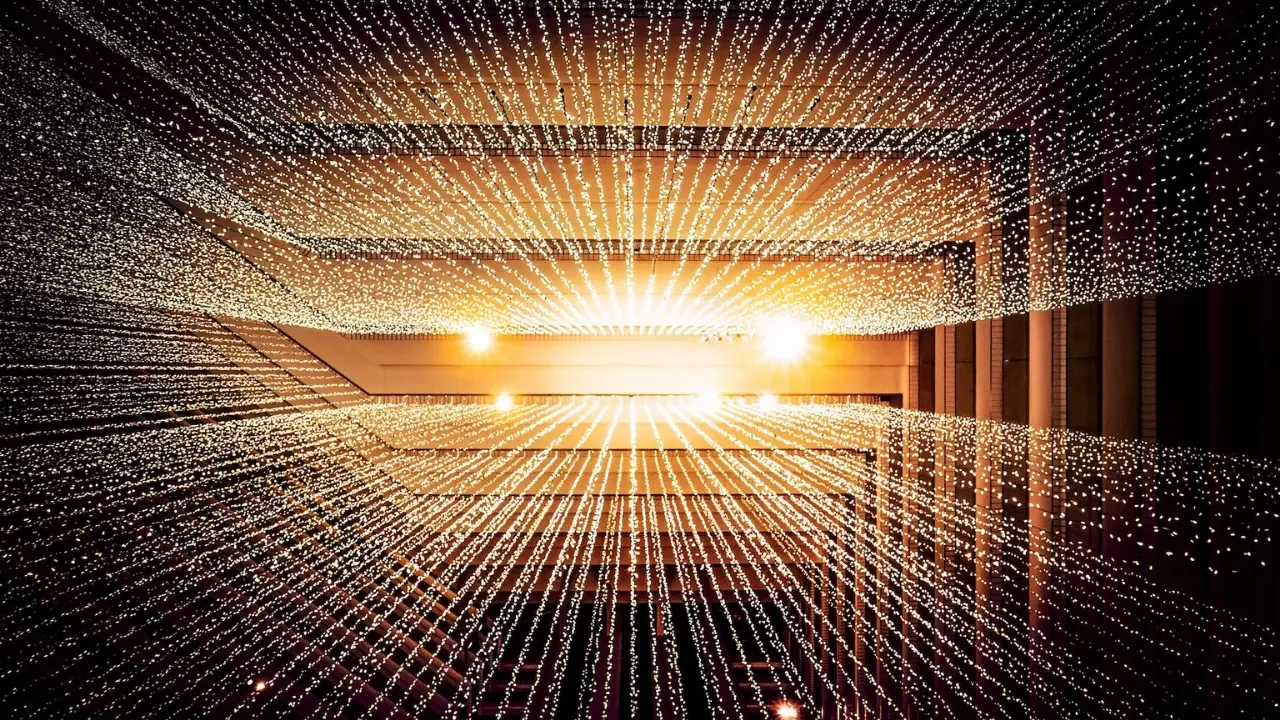
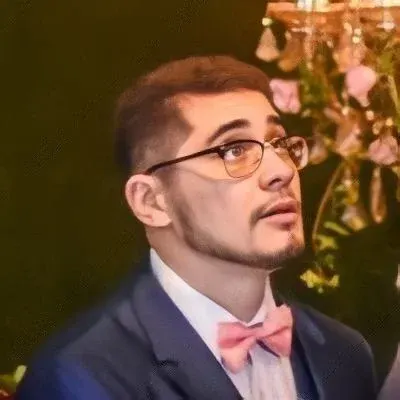
Quickly Reading Very Large Tables as Dataframes 💻💨
Are you struggling to load very large tables as dataframes in R? Do you find that the usual methods are slow and inefficient? 🐢💤 Don't worry, we've got you covered! In this blog post, we will address common issues when dealing with large tables and provide easy solutions to help you read those tables quickly and efficiently. So, let's dive in! 🏊♀️💥
The Challenge: Loading Large Tables 📈
You have a massive table with 30 million rows, and you want to load it as a dataframe in R. 🔄 However, using the read.table()
function, which is commonly used for this purpose, seems to slow things down due to its complex implementation logic. 😫
But fear not! We can leverage alternative approaches and optimize our code to achieve blazing-fast performance. 💪🚀
The Solution: Optimizing the Table Reading Process 🚀🔍
1. Using scan()
instead of read.table()
One way to improve the reading speed is by using the scan()
function instead of read.table()
. 📖 scan()
reads data directly and is faster for large tables. Here's an example of how you can use it:
datalist <- scan('myfile', sep='\t', list(url='', popularity=0, mintime=0, maxtime=0))
2. Converting the List to a Dataframe
Since we have the data in a list format, we need to convert it into a dataframe. However, this step can decrease the performance. 😞 To make it more efficient, use the unlist()
function in conjunction with as.data.frame()
, like this:
df <- as.data.frame(unlist(datalist))
3. Specify Column Types
To further optimize the process, it's essential to specify the column types before reading the table. This way, R won't have to infer the column types, resulting in faster execution. 💡 Here's an example:
df <- as.data.frame(unlist(datalist), stringsAsFactors = FALSE)
df$url <- as.character(df$url)
df$popularity <- as.integer(df$popularity)
df$mintime <- as.integer(df$mintime)
df$maxtime <- as.integer(df$maxtime)
By explicitly defining the column types, we eliminate unnecessary guesswork, thereby accelerating the process. ⚡️
4. Consider Parallel Processing
If your system supports it, you might consider leveraging parallel processing techniques to read and load the large table much faster. Parallelization distributes the workload across multiple cores, making the task more efficient. However, be aware of potential memory constraints when working with very large datasets. 🧠⚡️
The Call to Action: Share Your Experiences! 📣🗣
We hope these solutions make loading large tables as dataframes a breeze for you! Give them a try and let us know how they work for your use case. Have any other tips or tricks to share? We'd love to hear them! Leave a comment below and join the discussion. 👇🤔
And don't forget to share this blog post with your fellow data enthusiasts who might be struggling with the same challenge. Let's help everyone unlock the power of R without compromising on speed! 💪💻
Happy coding! 💃🎉