Call apply-like function on each row of dataframe with multiple arguments from each row
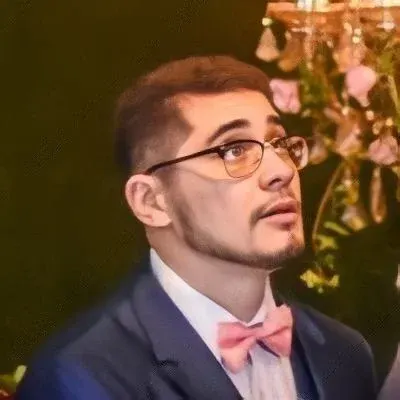
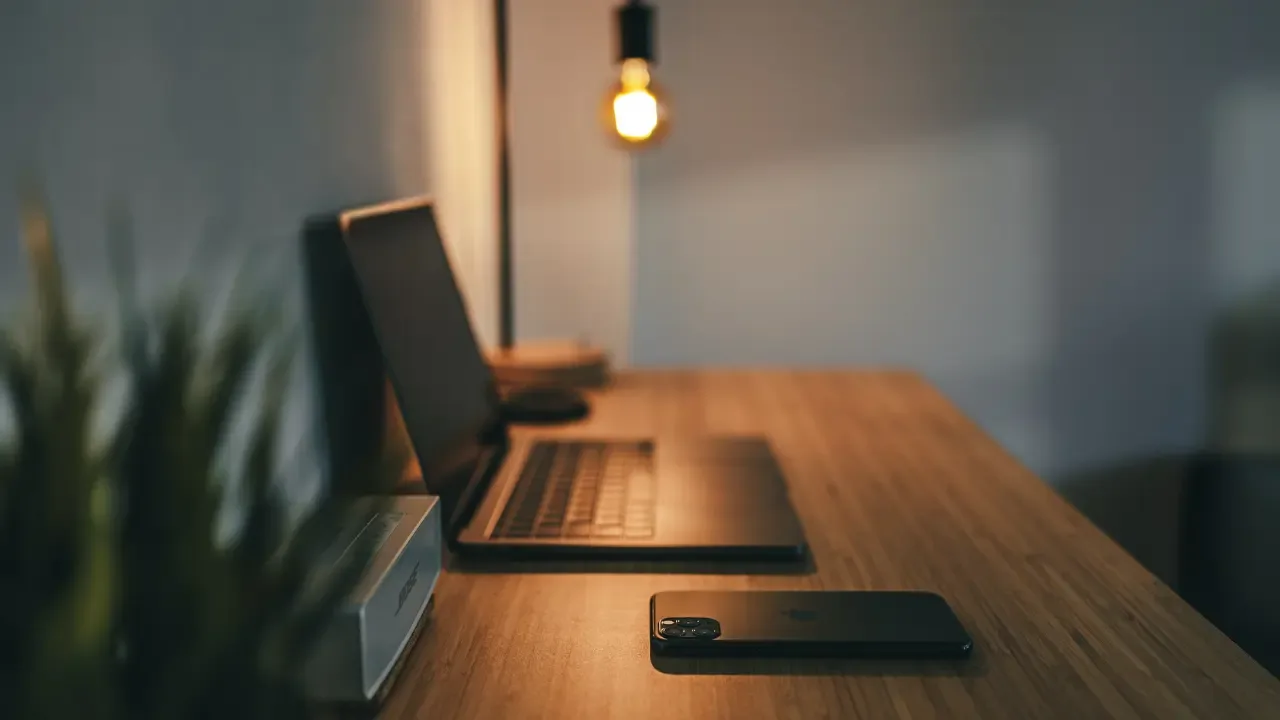
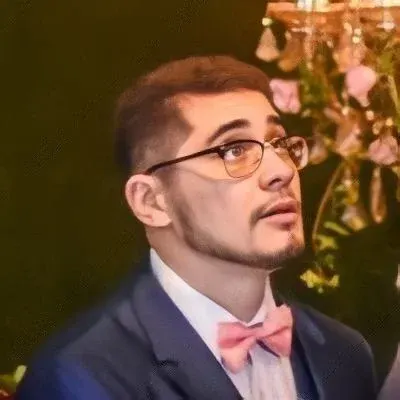
Applying a Function to Each Row of a DataFrame with Multiple Arguments
š Hey there! Are you trying to call a function on each row of a dataframe, using multiple arguments from each row? š§ It sounds like you're facing a common issue, but don't worry, I've got you covered! In this blog post, we'll explore how to tackle this problem and provide you with easy solutions. Let's dive in! šŖ
Understanding the Problem
So, you have a dataframe with multiple columns, and for each row, you want to apply a function. The catch is, your function requires multiple arguments, which you want to extract from each row of the dataframe. Let's consider the following scenario:
# Creating a dataframe
df <- data.frame(x = c(1, 2), y = c(3, 4), z = c(5, 6))
# Printing the dataframe
df
The output:
x y z
1 1 3 5
2 2 4 6
Now, let's say you have a function called testFunc
that accepts two arguments and you want to apply it using columns x
and z
as inputs. For example, you want to calculate x + z
for each row.
Approach with lapply
One way to achieve this without using a for loop is to leverage the lapply
function from the apply family. Here's what you tried initially:
# Selecting relevant columns
df_select <- df[, c('x', 'z')]
# Applying testFunc using lapply
lapply(df_select, testFunc)
Unfortunately, this resulted in an error: Error in a + b : 'b' is missing
. This error occurs because when lapply
applies the function testFunc
to each column of df_select
, it treats each column as a separate argument (a and b). However, testFunc
expects two arguments, and lapply
doesn't provide a way to pass multiple column values as arguments by default. Let's explore a solution to address this issue. š¤
Solution: Using mapply
or Map
To pass multiple arguments from each row, you can use either the mapply
or Map
functions. Both functions are part of the apply family and allow you to apply a function with multiple arguments to each column or vector of a dataframe.
Solution 1: mapply
The mapply
function applies a given function to the corresponding elements of one or more vectors or dataframes. In our case, we can utilize it like so:
# Applying testFunc using mapply
result <- mapply(testFunc, df_select[['x']], df_select[['z']])
This will return a vector with the output of testFunc
applied to each row. In our example, the result will be c(6, 8)
.
Solution 2: Map
Similar to mapply
, the Map
function applies a function to the corresponding elements of one or more vectors or dataframes. However, Map
does not simplify the result to a vector by default. Here's how you can use Map
to solve your problem:
# Applying testFunc using Map
result <- Map(testFunc, df_select[['x']], df_select[['z']], SIMPLIFY = FALSE)
This will return a list with the output of testFunc
applied to each row. In our example, the result will be a list: list(6, 8)
.
Real-Life Example: Applying power.t.test
to Each Row
Now that you understand the general approach, let's consider a real-life example. Suppose you have a dataframe called df
with columns delta
, power
, and sig.level
, and you want to apply the function power.t.test
to each row using the corresponding values as arguments. Here's how you can achieve this:
# Creating the dataframe
df <- data.frame(
delta = c(delta_values),
power = c(power_values),
sig.level = c(sig.level_values)
)
# Applying power.t.test using Map
result <- Map(
power.t.test,
df[['delta']],
df[['power']],
df[['sig.level']],
SIMPLIFY = FALSE
)
This will return a list where each element corresponds to the output of power.t.test
applied to each row of df
.
Conclusion
You've made it to the end! š We've explored how to apply a function to each row of a dataframe, utilizing multiple arguments from each row. We discussed two solutions using the mapply
and Map
functions, plus a real-life example using power.t.test
. Hopefully, these solutions help alleviate any headaches you had. Now go forth and apply functions to your heart's content! šŖš»
If you found this blog post helpful, feel free to share it with your fellow data enthusiasts! And if you have any questions or other tricky problems you'd like us to tackle, drop a comment below. Let's keep learning and coding together! š©āš»šØāš»