Tricks to manage the available memory in an R session
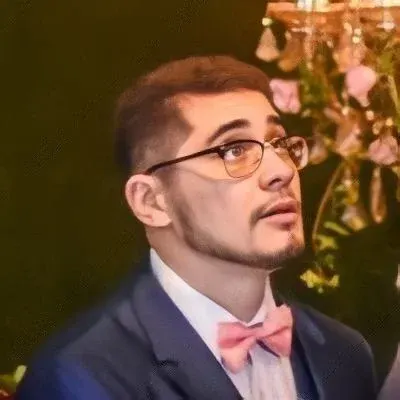
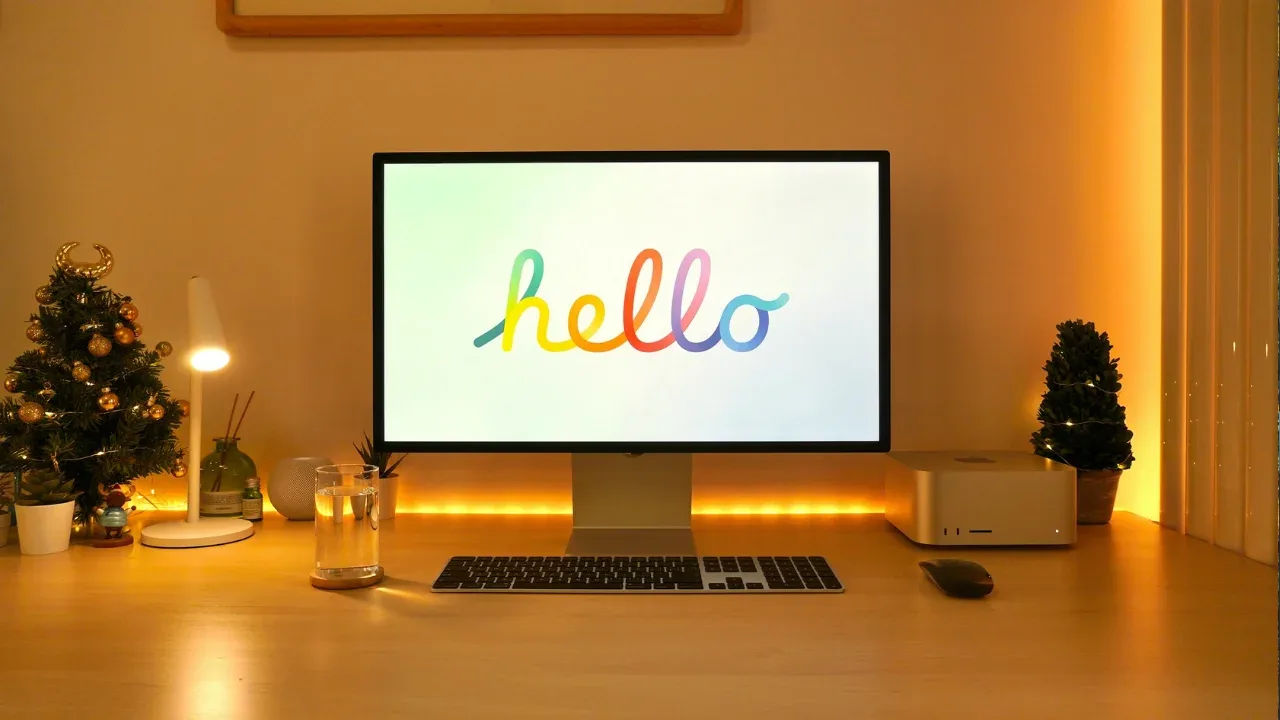
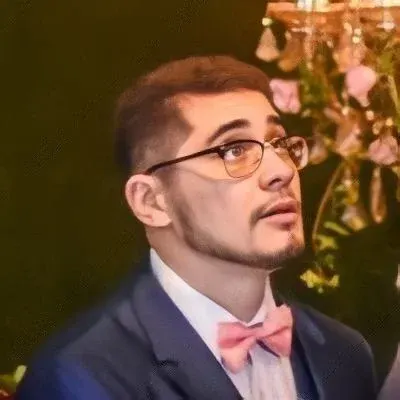
š Tricks to manage the available memory in an R session
šāāļø Are you facing issues with managing memory in your interactive R session? No worries, we've got you covered! In this blog post, we'll share some easy tricks to help you efficiently handle the available memory in your R sessions.
š¤ First, let's understand the problem at hand. When working with large datasets or complex calculations, R sessions can consume a significant amount of memory, leading to performance issues or even crashing. It's essential to optimize your memory usage to keep your R session running smoothly.
š” To get started, let's look at a couple of functions that can help us identify and handle memory-consuming objects:
# improved list of objects
.ls.objects <- function (pos = 1, pattern, order.by,
decreasing=FALSE, head=FALSE, n=5) {
napply <- function(names, fn) sapply(names, function(x)
fn(get(x, pos = pos)))
names <- ls(pos = pos, pattern = pattern)
obj.class <- napply(names, function(x) as.character(class(x))[1])
obj.mode <- napply(names, mode)
obj.type <- ifelse(is.na(obj.class), obj.mode, obj.class)
obj.size <- napply(names, object.size)
obj.dim <- t(napply(names, function(x)
as.numeric(dim(x))[1:2]))
vec <- is.na(obj.dim)[, 1] & (obj.type != "function")
obj.dim[vec, 1] <- napply(names, length)[vec]
out <- data.frame(obj.type, obj.size, obj.dim)
names(out) <- c("Type", "Size", "Rows", "Columns")
if (!missing(order.by))
out <- out[order(out[[order.by]], decreasing=decreasing), ]
if (head)
out <- head(out, n)
out
}
# shorthand
lsos <- function(..., n=10) {
.ls.objects(..., order.by="Size", decreasing=TRUE, head=TRUE, n=n)
}
š The code above provides an improved list of objects and a shorthand function to easily access the largest objects in terms of size. Let's break it down:
The
.ls.objects
function helps us list and sort the largest objects in our R session. It takes parameters likepos
,pattern
,order.by
,decreasing
,head
, andn
, which allow us to customize the output based on our requirements.The
lsos
function acts as a shorthand for.ls.objects
and provides the top "n" largest objects by size. You can tweak the value ofn
as per your needs.
š Now, let's explore some tricks to manage memory effectively:
Identify the memory hogs - Use the
.ls.objects
orlsos
functions to identify the largest objects in your R session. By understanding which objects consume the most memory, you can prioritize memory optimization efforts.Remove unnecessary objects - Once you've identified the memory-consuming objects, you can use the
rm()
function to remove them from your R session. Be cautious and double-check that you no longer need those objects before deleting them.Avoid loading unnecessary libraries - Loading unnecessary libraries can occupy memory resources. Only load the specific libraries required for your current task to reduce memory usage.
Optimize data structures - Choose the appropriate data structures for your data. For example, if you have a large dataset that doesn't require modification, consider using read-only data frames (
data.table
ordplyr
).Use memory-efficient alternatives - Some packages provide memory-efficient versions of commonly used functions. For example, the
data.table
package offers memory-efficient alternatives to base R functions likemerge()
andsubset()
.Split data into smaller chunks - If your dataset is too large to fit in memory, consider splitting it into smaller chunks and process each chunk individually. You can then combine the results later.
Avoid unnecessary copying - Be mindful of unnecessary copying of objects in your code. Assigning objects using
=
or<-
creates new copies, consuming additional memory. Instead, modify objects in-place whenever possible.
šŖ Implementing these memory management tricks will help you optimize your R sessions, reduce memory usage, and improve performance.
š Have any other useful tricks for managing memory in an R session? Join the conversation by leaving a comment below! Let's learn from each other and make our R sessions more efficient together.
š Don't forget to hit the share button and spread the word. Happy coding! šāØ